Mastering Video Annotation: Unlocking the Potential of Your Data
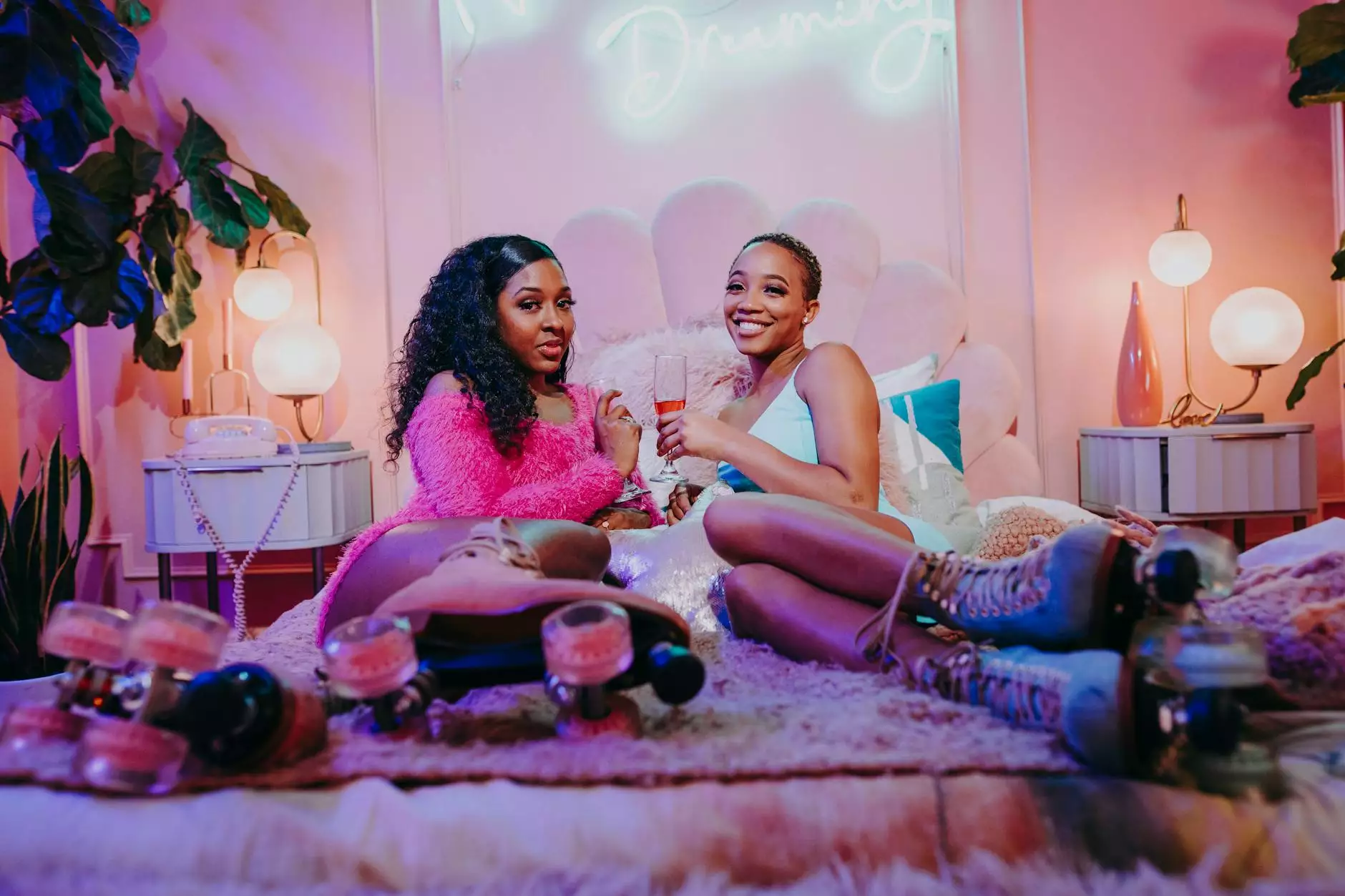
Understanding Video Annotation
Video annotation is the process of labeling and tagging video content to provide useful data for machine learning and artificial intelligence models. This technique is crucial for training algorithms to analyze visual information, allowing them to understand contexts, objects, events, and scenes within a video stream. As the demand for video content grows across industries—from security surveillance to autonomous vehicles—so does the need for effective data annotation tools.
Why Video Annotation is Important
The significance of video annotation cannot be overstated. In a world increasingly dominated by visual data, accurate labeling of videos creates a robust foundation for various applications. Here are a few key reasons why it is essential:
- Enhances Machine Learning Models: Properly annotated video data helps in enhancing the accuracy of machine learning models, making them more reliable.
- Facilitates Object Detection: Video annotation allows for the identification of objects and activities, paving the way for advanced applications in sectors like retail and security.
- Improves User Experience: By enabling personalized content recommendations and better search functionalities, video annotation significantly improves user engagement.
- Powers Automation: Industries like transportation and surveillance use video annotation to automate processes, increasing efficiency.
How Keylabs.ai Transforms Video Annotation
Keylabs.ai stands at the forefront of data annotation platforms, providing comprehensive solutions tailored for video content. This innovative platform offers a suite of tools designed to streamline the video annotation process, ensuring high-quality outputs with minimal manual effort.
Features of Keylabs.ai's Video Annotation Tools
Keylabs.ai's platform is equipped with numerous features that set it apart from others. Let’s dive deeper into what makes it a preferred choice for businesses:
- Automated Annotation: The integration of AI-driven automated annotation capabilities drastically reduces the time and effort required for video labeling.
- User-Friendly Interface: The intuitive interface simplifies the annotation process, making it accessible even for users with minimal technical skills.
- Real-Time Collaboration: Teams can work collaboratively on the same video project, enhancing productivity and ensuring consistency in data quality.
- Customizable Workflows: Users can tailor workflows to meet specific project requirements, making the annotation process adaptable to various industries.
- Quality Assurance Tools: Built-in quality check features ensure that annotated data meets the highest standards before it is applied to machine learning models.
The Process of Video Annotation
Understanding the process of video annotation is crucial for maximizing its benefits. Here's a step-by-step outline of how the process typically unfolds:
1. Video Selection
The first step involves selecting relevant video footage that needs to be annotated. The choice of video is critical, as it should align with the goals of the machine learning model.
2. Setting Annotation Parameters
Defining parameters such as object types, events to monitor, and specific labeling criteria is essential in producing tag data that enhances model training.
3. Annotation Execution
This is where the actual annotation happens, using tools to label objects, actions, and context within the video. Tools provided by platforms like Keylabs.ai make this both precise and efficient.
4. Quality Review
Quality assurance is paramount. This step includes a second round of checks where the annotations are reviewed for accuracy, making necessary corrections to ensure the highest quality of data.
5. Data Export
Once the annotations are verified, the data can be exported in various formats (such as JSON or XML) suitable for feeding into machine learning models.
Applications of Video Annotation
The versatility of video annotation allows it to be used across various sectors. Below are some significant applications:
- Autonomous Vehicles: Video footage from vehicles is annotated for object detection, helping self-driving cars recognize pedestrians, road signs, and other vehicles.
- Surveillance: In security applications, video annotation aids in identifying suspicious activities or behaviors in real-time.
- Health Care: Medical imaging data can be annotated for video analysis in surgeries or diagnostic procedures, improving patient outcomes.
- Sports Analytics: Coaches and analysts use annotated videos to dissect player performances and strategize better.
- Retail Analytics: Businesses analyze customer behaviors in stores through annotated videos, enhancing customer experience and increasing sales.
Challenges in Video Annotation
While video annotation presents significant advantages, it is not without its challenges. Here are some of the main hurdles businesses face:
- Time-Consuming: Manual annotation can be tedious and time-intensive, especially for long video clips.
- Quality Control: Maintaining consistency and accuracy during annotation is vital, but challenging without proper checks.
- Training Required: Annotators need to understand the specific requirements for each project, which may necessitate considerable training.
- Data Privacy: Ensuring that sensitive information is handled appropriately can complicate the annotation process.
Best Practices for Video Annotation
To maximize the effectiveness of your video annotation processes, consider the following best practices:
- Define Clear Objectives: Set specific goals for your annotation project to guide the team and maintain focus.
- Invest in Training: Equip your annotators with the knowledge and tools needed to execute annotations accurately.
- Utilize Advanced Tools: Leverage platforms like Keylabs.ai that provide robust features to improve productivity and accuracy.
- Implement Regular Audits: Conduct frequent quality checks to ensure the annotated data meets the desired standards.
- Maintain Documentation: Keep clear records of labeling guidelines and changes in annotation practices to streamline future projects.
Looking Ahead: The Future of Video Annotation
The field of video annotation is evolving rapidly with advancements in AI and technology. Future trends likely to shape the sector include:
- Greater Automation: Expect more sophisticated AI tools that can perform annotations automatically with increased accuracy.
- Enhanced User Interfaces: Continual improvements in usability will streamline the annotation process even further.
- Integration of Augmented Reality: AR could play a role in providing real-time annotations during video recordings.
- Ethical AI Considerations: As data privacy becomes more paramount, ethical considerations will shape annotation practices.
Conclusion
In conclusion, video annotation is a powerful tool that unlocks vast potential for businesses leveraging data-driven solutions. With platforms like Keylabs.ai, organizations can automate and optimize their video processing, leading to enhanced machine learning applications and improved operational efficiencies. By understanding the best practices and embracing emerging trends, companies can position themselves ahead in the competitive landscape, maximizing the value derived from their video data.